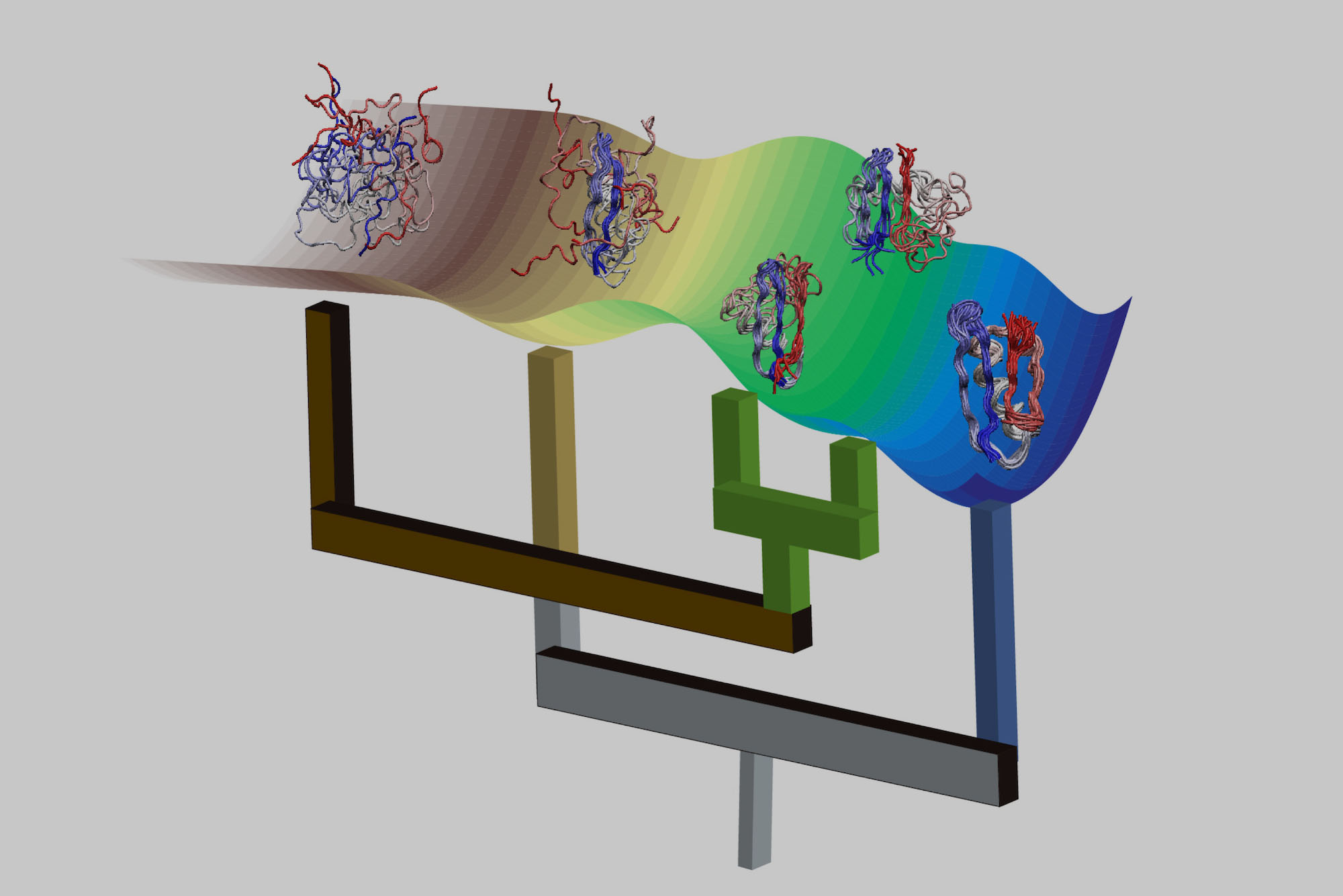
Using AI, UF Chemists Chart Molecules’ Most Important Shapes
New algorithms reveal which molecular states matter most for disease progression and drug design
The molecules that make up the human body don’t make it easy on chemists trying to understand their behaviors. Each can take on a variety of complex states that affect how diseases progress, how well drugs work, and many other important biological processes.
Through innovative techniques that draw upon artificial intelligence and machine learning, University of Florida computational chemists are working to solve the mystery of these variations to give researchers a deeper understanding of our bodies’ molecules in all their shapes and forms.
To do this, UF chemists developed new algorithms that can efficiently find previously unknown similarities among the different shapes of molecules produced by living organisms, such as proteins and DNA. The approach, introduced in the journal Physical Chemistry Chemical Physics (PCCP), will help to reveal biomolecules’ most important states and open new pathways for intervention against diseases. It could also lower hurdles and quicken the pace in the drug discovery process.
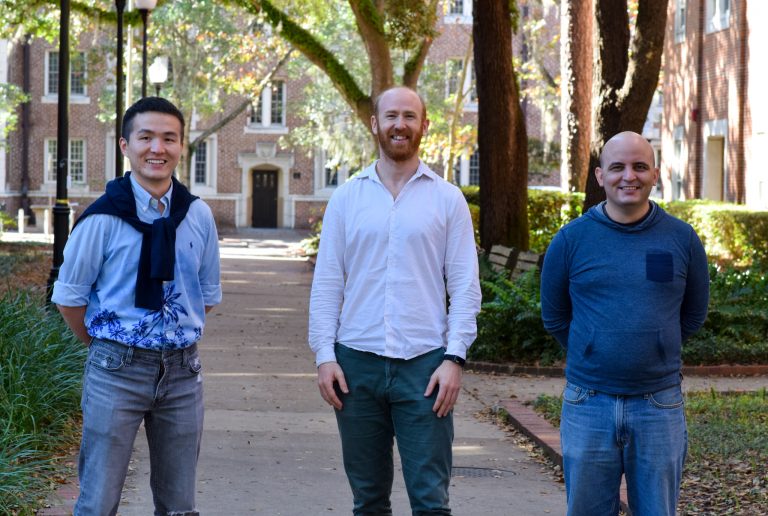
“This is a very powerful tool, because by identifying these key states we can then target them with tailor-made drugs and inhibitors,” said RAMON A. MIRANDA QUINTANA, PhD, assistant professor of chemistry and one of the paper’s authors. Miranda Quintana worked with ALBERTO PEREZ, PhD, assistant professor of chemistry, and graduate student LIWEI CHANG (all members of UF’s Quantum Theory Project) to develop the new method.
Chemists have long had to rely on complex, time-consuming methods to analyze variations in molecular states, slowing the development of targeted treatments for disease. The traditional methods involved directly comparing molecular states to each other one-on-one to identify relationships within the pairs. The UF researchers wanted a broader view: By teaching their algorithms to simultaneously compare thousands of different states, they bring to light similarities that wouldn’t be noticed by the usual techniques.
To realize the potential of these new tools, the chemists are looking to collaborators across UF’s campus. In joint work with Chenglong Li, PhD, and Gustavo Seabra, PhD, of the College of Pharmacy, the researchers will apply their methods to drug design, incorporating the algorithms into an artificial intelligence-driven drug discovery platform.
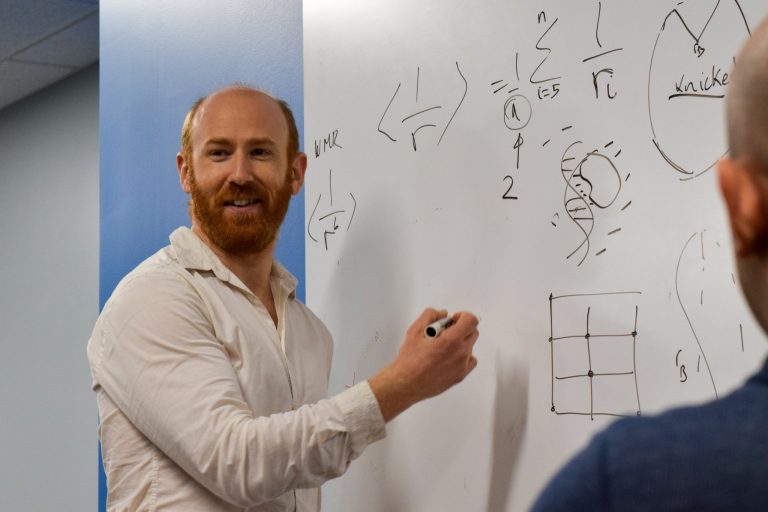
The team is also working with fellow UF chemist BOONE M. PRENTICE, PhD, whose use of mass spectrometry imaging — a technique that visualizes the spatial distribution of molecules within tissues — generates massive amounts of data. The algorithms can quickly sift through this mountain of information to better understand the progression of diseases like Type 1 diabetes.
The researchers made the algorithms as broadly applicable as possible, allowing them to examine a wide variety of molecular behaviors and interventions. They’ve already been able to identify key structures involved in protein folding — a process that allows a protein to perform its function — that other methods had a very hard time finding, demanding a much higher computational cost. The team expects that the algorithms will bring more relationships to light and lead to further discoveries.
“This information is key in the biomedical sciences, since it will improve our understanding of diseases at a molecular level, leading to the design of more potent and selective treatments,” Miranda Quintana said.
Ready the full study in PCCP here.